Home | Download this report (PDF) | Survey methodology and topline
March 12, 2025
The rise of large language models has been historic. In less than two-and-and-half years, half the adults in America say they have used LLMs. Few, if any, communications and general technologies have seen this pace of growth across the entire population.
A surging wave of adoption in the past year has been added to the early adopters. It is now the case that the share of women using LLMs is the same as that of men. Notably higher shares of Hispanic adults and Black adults use LLMs, compared with White adults. There are strikingly small differences among those with different levels of household income and those with different levels of educational attainment. Those who work full-time (62%) and part-time (55%) are relatively likely to use LLMs. Yet, it is also true that 41% who are not employed – such as retirees, homemakers and those with disabilities – use the models.
There are some interesting differences among different classes of workers: High-level executives, science professionals and licensed professionals such as educators and even junior managers are particularly likely to use LLMs. Still, these other groups are LLM users: 62% of frontline retail and service workers such as store clerks and restaurant wait-staff, 56% of junior-level clerical workers and 43% of skilled farmers and factory workers/
Notably, households with minor children (61%) are more likely than those without minors (50%) to be LLM users.
Two other significant developments have occurred since LLMs were launched in a friendly interface in ChatGPT: The first is the explosive growth of specialized LLMs that focus on specific subjects like law, chemistry or climate issues. When it comes to people’s use of specialized models among these LLM users, we found:
- 80% of LLM users have tried special models that help them do research.
- 75% have tried special models that help them write.
- 67% have tried special models that help them with tutoring and learning.
- 66% have tried special models that help them pursue lifestyle and hobby activities.
- 59% have tried special models that help them design creations.
- 42% have tried special models that help them with computer coding.
The second way LLMs are spreading is the inclusion of LLM bots and tools in commonly used digital applications such as email, document creation, and social media apps. The fact that LLMs are now incorporated in common applications suggests that the total universe of LLM users is larger than we captured in our overview because people might be using LLMs without knowing the tools are employed in the everyday products they use. Our questions about LLM use in existing digital applications found:
- 61% of these LLM users have used language model applications inserted into social media platforms.
- 58% have used LLM apps inserted into text messaging systems.
- 58% have used LLM apps inserted into photo-editing software.
- 51% have used LLM apps inserted into email programs.
- 47% have used LLM apps inserted into video conferencing software such as Zoom.
- 41% have used LLM apps inserted into word-processing programs.
- 38% have used LLM apps inserted into presentation software such as PowerPoint.
- 36% have used LLM apps inserted into graphic design software.
- 34% have used LLM apps inserted into spreadsheets.
- 25% have used LLM apps inserted into project management or collaboration tools such as Slack.
The language models they use
ChatGPT is the most commonly used LLM, followed by Gemini. Some 58% of LLM users have tried two or more models. ChatGPT also stands out as the LLM that people use the most.
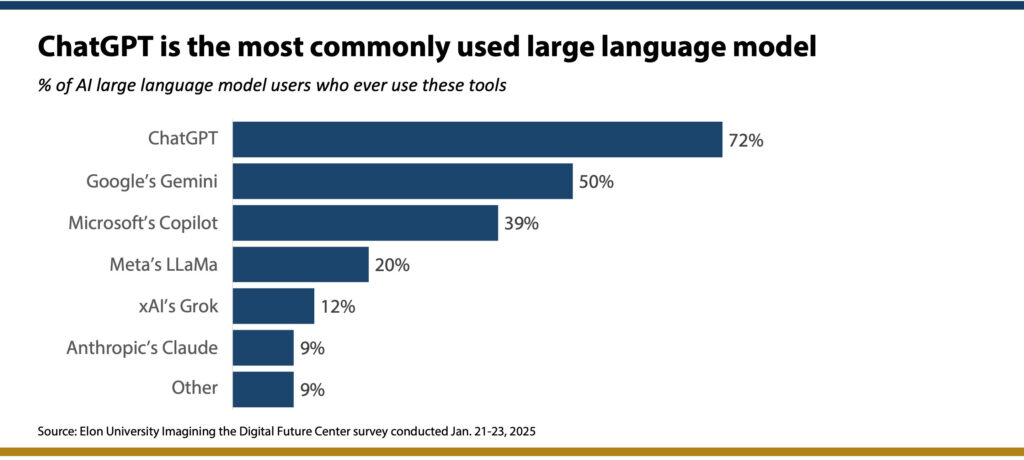
When asked if they have a paid subscription for the LLMs they use, 20% said they do, and most of those using paid-for models have the subscription costs covered by another party rather than themselves. Just 4% of LLM users say they pay for a subscription themselves. Fully 80% of model users say they use the free versions.
We also asked these LLM users if they had ever used AI image generators such as DALL-E, Midjourney, Adobe Firefly or ImageFX. Fully 67% of LLM users said they also have used such image generators: 18% said they did so at least once a day or more often; 12% said they had done so several times a week and 36% said they had used image generators less often than that.
How and why people use LLMs
Much of the focus on the rise of LLMs has been on people’s use of the models in workplaces. This survey finds that when a probability sample of the whole adult population is canvassed, people are far more likely to say that personal uses are more common than work-related uses. Among a range of possible uses, more people cite personal uses like informal learning and personal amusement than cite work activities. When they are asked about the main purpose they use LLMs, twice as many say personal, informal learning and planning (51%) than say work activities (24%).
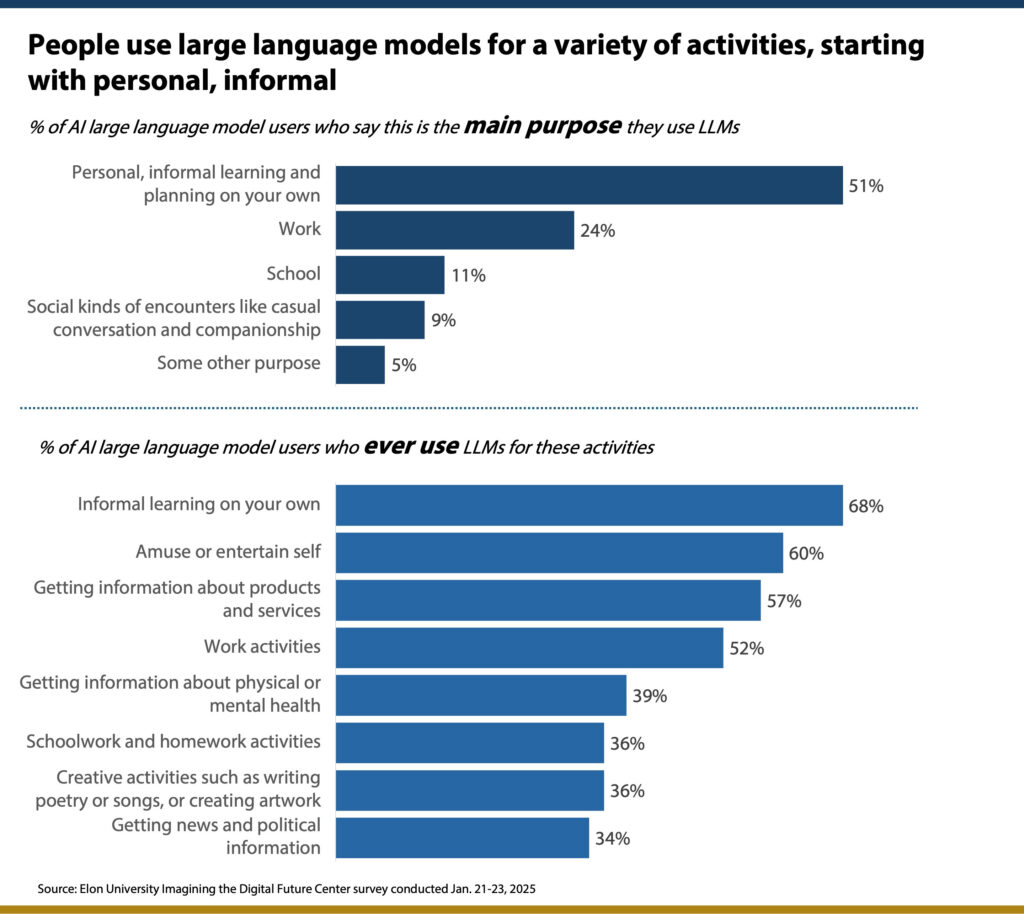
There are serious implications in these findings. Among other things, they show the degree to which LLMs are now being used in the way that people have used search engines for decades, including quick access to information, queries about products and services and getting news and information. This has enormous implications for media, marketing and the basic sale of goods and services. It also suggests the profound impact LLMs might have on political and civic processes. Moreover, they suggest that for segments of the LLM-user cohort, the models are being incorporated into their basic learning and exploration activities and the creative process. Here are some of the demographic insights these findings generate:
Informal learning on their own: This is particularly true for the LLM users who are men.
Amuse or entertain themselves: This is especially true for men and LLM users without college degrees.
Information about products and services: This is particularly true for men who are LLM users and those ages 55 and older.
Work activities: LLM users under age 55, those who live in higher-income households, and those with college degrees are more likely to pursue these activities.
Physical and mental health: Adults under age 65 and those in households earning less than $50,000 are particularly likely to use LLMs.
Creative activities such as writing poetry or songs or creative artwork: This use of LLMs is especially popular with users under age 55 and those living in households earning less than $100,000.
Schoolwork and homework: This is especially true for the LLM users under age 55 and those who live in a household earning less than $50,000.
Political news and information: The LLM users who are Republicans are more likely than Democrats to use the models this way, as are those who do not have college degrees.
The survey also covered a dozen other specific possible uses of LLMs. The responses show some of the diverse purposes that LLMs are serving, ranging from using them like search engines and for researching new subjects to planning social gatherings and even checking up on what the models say about them.
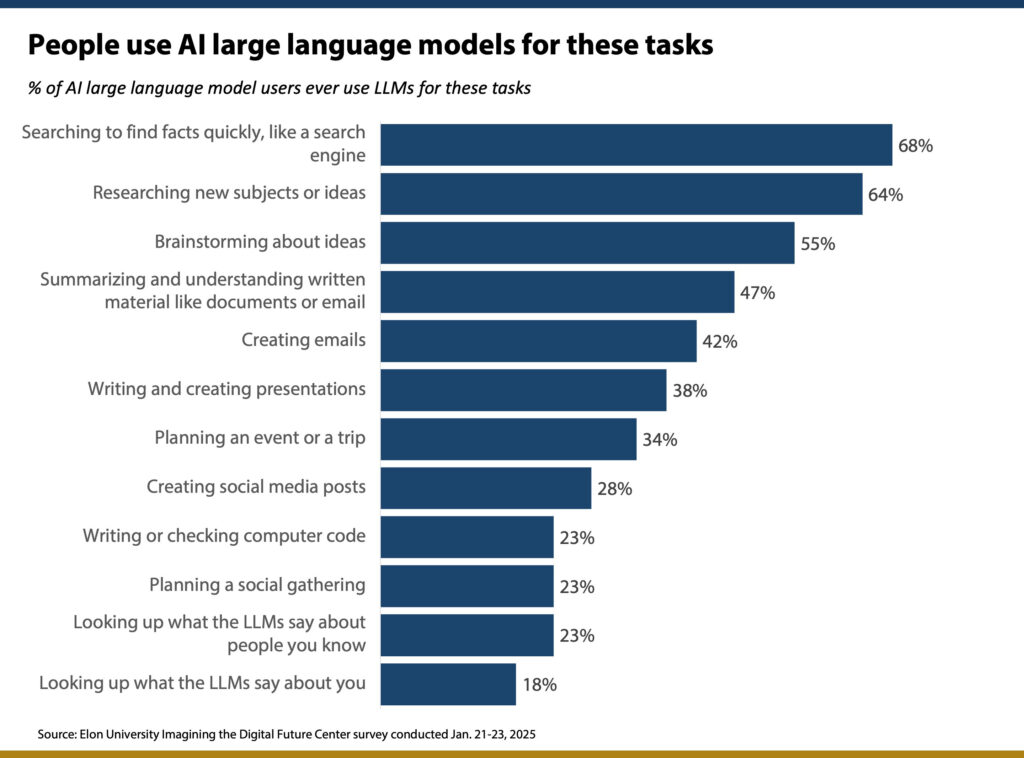
Some interesting demographic differences exist in some of the activities queried here. They include:
- By gender: Men who are LLM users are more likely than women to use the models to research new subjects or ideas. On the other hand, women who use LLMs are more likely than men to use the models to plan an event or trip and to plan a social gathering.
- By education: Those with college degrees are more likely than those who ended their education at high school or less to use LLMs for researching new subjects or ideas and for writing and creating presentations. In contrast, those with high school or less are more likely than those with college degrees to use LLMs to create social media posts.
- By race and ethnicity: Comparing White LLM users with non-White LLM users, non-White users are more likely to use the models to summarize and understand material like documents or emails; to use the models to write or check computer code; to write social media posts and to look up what LLMs say about people they know.
- By age: Younger LLM users are more likely than older users to employ LLMs to write code.
The traits of language models – intelligence and conversation
Those who use LLMs experience the models in a wide range of encounters and think the models seem to show diverse reactions and stances toward them. This illustrates the degree to which these generative AI tools represent new ways for people to experience and exploit technology – and also new ways for technology to mimic, exploit and even surpass human-like “behaviors” with people. These findings have special relevance as model builders feverishly rush to create AI agents that they hope to promote as entities that can act on their users’ behalf and represent their users.
LLM systems are often benchmarked against standard human intelligence tests. The race to create human-level artificial general intelligence (AGI) is the driving force behind big tech firms’ development of LLM systems.
In this survey, LLM users were asked about this, and about half (49%) said they thought the models were at least a little smarter than they are. A quarter said the models they use are a lot smarter than they are; another 23% said the models are a little smarter and 18% said the models are about as smart as they are. Women who use LLMs were more likely than men to think the models are a lot smarter (30% vs. 20%). The LLM users with college degrees were more likely than those with less education to say the models are a lot less smart than they are.
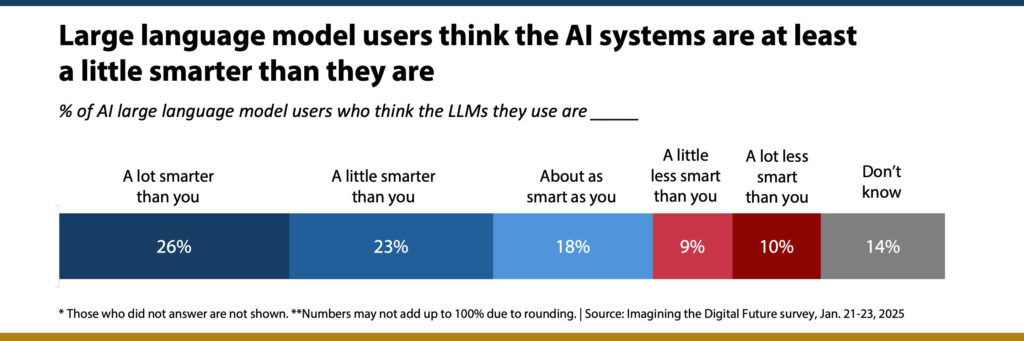
Another dramatic example of how LLMs show human-like traits is their capacity to be involved in conversation and social interaction with users. The survey shows that 65% of LLM users have had a back-and-forth discussion with an LLM where they used their voice and the model replied in a realistic voice. Their demographic profile is significant: 76% of the LLM users in households earning less than $50,000 have conversations with the models and 83% of the non-White users have such conversations.
A third of LLM users can be viewed as “regular conversation users” – they say they have conversations with LLMs almost constantly (3%), several times a day (11%), about once a day (6%) or several times a week (13%). Another 31% say they have conversations less often than that. Those regular conversation users are especially likely to be ages 18-34, in lower-income households and hold a high school diploma or less. These regular conversation users stand out from other LLM users in a host of ways for their attachments to LLMs and the use of LLMs (see section “The special habits and views of LLM conversation users” below).
This survey sought to capture model-user views across several traits. Users were the most enthusiastic about the professional-looking traits. They were generally more likely to say the models exhibited positive traits like humor, curiosity, hope, and empathy than negative traits like jealousy, anger, sadness and sarcasm.
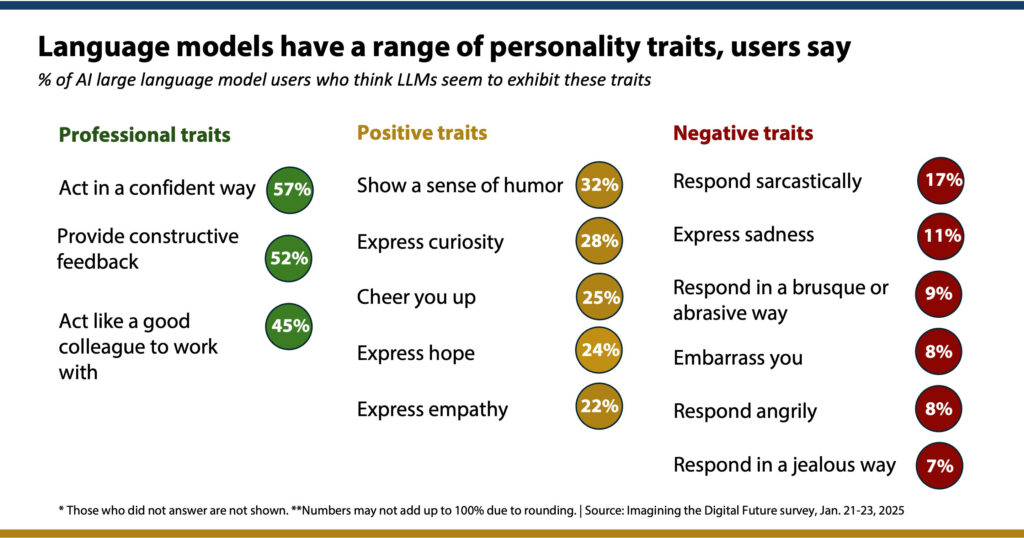
Interestingly, the demographic data on these questions do not show strikingly different patterns. People of all groups of LLM users tend to see these personality traits in the same way.
We also asked about some possible attributes of the models on some kinds of social behavior and social learning activities that are possible with LLMs. About half of model users (53%) say the LLMs they use give personalized answers to their prompts much of the time or some of the time. In addition, 43% say the models learn from their encounters with them much/some of the time. More strikingly, shares of model users say LLMs show the capacity to think and reason, to understand the user and make moral judgments about right and wrong. In all, these examples, notable shares say LLMs “at least a small amount of the time” show each attribute.
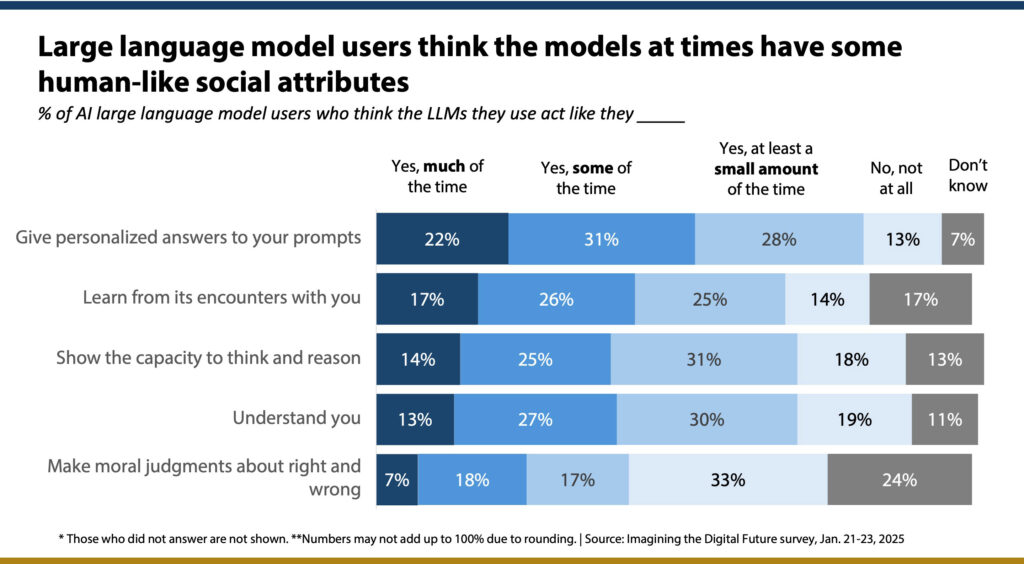
How LLMs perform for users and affect them
In all, 76% of LLM users say they are very satisfied (25%) or somewhat satisfied (50%) with the overall performance of the models. Just 11% are not too satisfied and 4% are not at all satisfied.
Young adults ages 18-34 are particularly likely to express satisfaction – 81% are very/somewhat satisfied. Otherwise, there are no notable demographic differences.
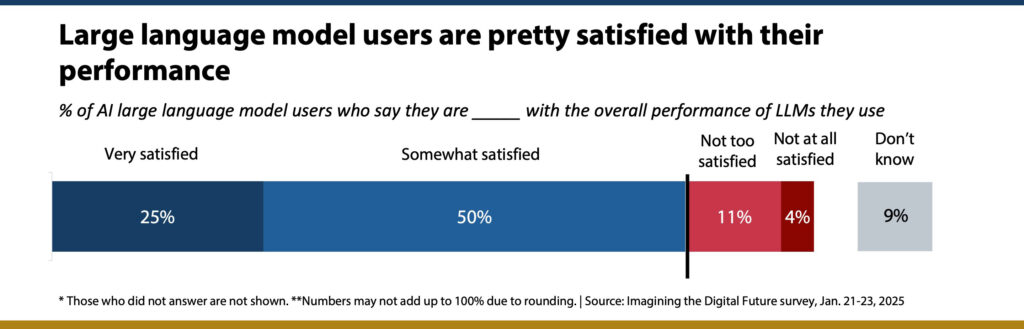
Unpacking that issue a bit, we asked about certain dimensions of LLMs’ performance and found majorities of users were very or somewhat satisfied with the models’ accuracy, the completeness of the answers they get and the fairness of the models. Additionally, 47% said they were very/somewhat satisfied with the models’ care in following ethics, compared with 25% who said they were not too satisfied or not at all satisfied.
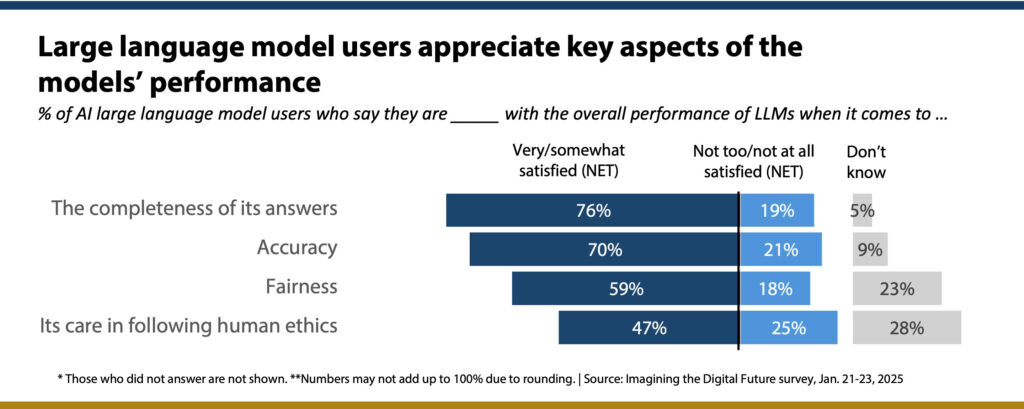
One demographic difference worth noting: 74% of the women who use LLMs are satisfied with the models’ accuracy, compared with 65% of LLM users who are men.
We also used several different lines of questioning to assess LLMs’ impact on users. One batch of questions looked at some of the possible ways LLM use might affect some practical aspects of everyday life. Modest majorities reported that their use of the models improved their productivity a lot or somewhat, lessened the amount of time they spend on annoying activities, and improved their ability to learn new skills and concepts. In addition, about 4-in-10 reported some level of improvement in their problem-solving skills, their creativity and their communications skills thanks to their use of LLMs.
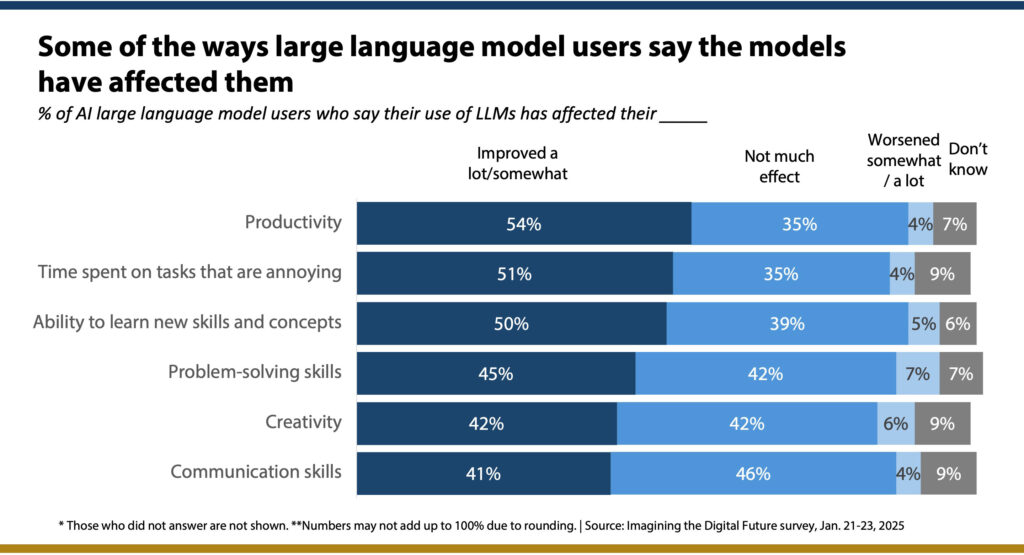
LLM users with college degrees were particularly likely to report that their LLM use had improved their productivity and lessened the time they spend on annoying tasks. Those without college degrees were especially likely to report that their LLM use had improved their ability to learn new skills and concepts and their creativity. Non-White users were more likely than White users to say their use of the models improved their communication skills and their problem-solving abilities.
Significant shares of LLM users also report that they have turned to the models when they were seeking help on major life moments:
- 41% of LLM users reported they had gotten help from an LLM getting training or more education to upgrade their job skills: 12% said the LLM had played a major role in helping them and the rest said the LLM played a minor role.
- 37% reported getting help from the LLM treating an illness or medical condition: 9% said the LLM played a major role.
- 28% said they had gotten help seeking or changing jobs: 9% said the LLM played a major role.
- 25% reported getting help making a major investment or financial decision: 7% said the LLM played a major role.
- 18% said they had gotten help deciding where to live: 6% said the LLM played a major role.
Beyond those uses, notable shares of LLM users also report a number of negative experiences with the LLM systems: Half say they felt at least at times like they were lazy or taking a too-easy shortcut in using the systems; a third felt they were becoming too dependent on LLMs to give them answers rather than thinking things through for themselves; and a third felt they were cheating and felt frustrated or confused as they used the models.
Some 23% said a lot of the time or sometimes they had made a significant mistake or bad decision when they relied on wrong information from an LLM. Around a fifth of the users also said they felt manipulated by an LLM and a similar share felt their use of an LLM had compromised their privacy. Some users also reported that their emotional and physical health had been hurt or that they had embarrassed themselves in a shameful way by relying on the LLM. For all of these questions there were additional respondents who said they had felt that way “but not very often.”
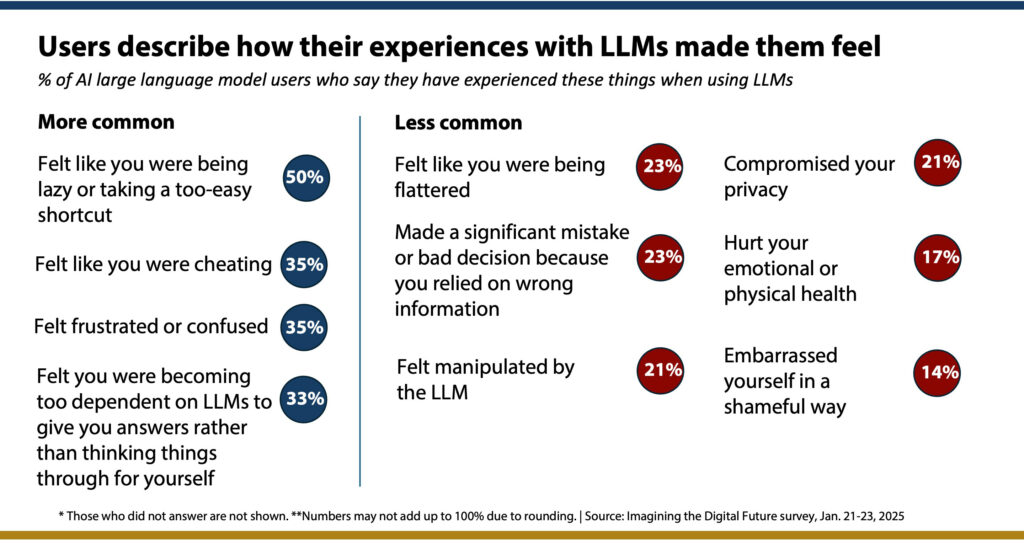
The special habits and views of regular LLM conversation users
As noted above, the 34% of LLM users who have human-like conversations with the models stand out in almost every way from other kinds of model users. Here are some of the main differences between regular LLM conversation users and those who do not have conversations with the models:
Usage: 84% of regular LLM conversation users use the models at least several times a week vs. 33% of those who never converse with LLMs.
- 82% of regular conversation users use the models for informal learning on their own vs. 58% of those who never converse.
- 75% of regular conversation users use the models for getting information about products and services vs. 37% of those who never converse.
- 67% of regular conversation users use the models to amuse or entertain themselves vs. 48% of those who never converse.
- 63% of regular conversation users use the models for work activities vs. 45% of those who never converse.
- 58% of regular conversation users use the models for getting information about physical or mental health vs. 21% of those who never converse.
- 55% of regular conversation users use the models for getting news and political information vs. 12% of those who never converse.
- 53% of regular conversation users use the models for creative purposes such as writing poetry or songs vs. 30% of those who never converse.
These regular conversation users are also far more likely than non-conversers to use models to write and create presentations (63% vs. 24%), brainstorm about ideas (73% vs. 46%), summarize and understand written material (77% vs. 23%), write and check computer code (47% vs. 10%), research new subjects or ideas (78% vs. 50%), create emails (62% vs. 32%), create social media posts (47% vs. 12%), plan an event (48% vs. 19%), plan a social gathering (38% vs. 8%), look up what LLMs say about people they know (43% vs. 4%) and look up what LLMs say about them (30% vs. 5%).
Impacts: 83% of regular conversation users say they are very or somewhat satisfied with the performance of LLMs they have used vs. 70% of those who never converse.
- 75% of regular conversation users are very/somewhat satisfied with the accuracy of the models vs. 56% of those who never converse.
- 62% of regular conversation users are very/somewhat satisfied with the fairness of the models vs. 45% of those who never converse.
- 58% of regular conversation users are very/somewhat satisfied with the models’ care in following human ethics vs. 26% of those who never converse.
- There were no differences between the two groups when it comes to the completeness of the answers LLMs delivers.
Attributes
- 87% of regular conversation users feel their primary LLM acts like it gives personalized answers to their prompts vs. 70% of those who never converse.
- 82% of regular conversation users feel their primary LLM acts like it learns from its encounters with them vs. 52% of those who never converse.
- 82% of regular conversation users feel their primary LLM acts like it understands them vs. 53% of those who never converse.
- 79% of regular conversation users feel their primary LLM acts like it shows the capacity to think and reason vs. 51% of those who never converse.
These regular conversation users are also far more likely than non-conversers to say the main LLM they use seems to cheer them up, provides constructive feedback, acts like a good colleague, expresses curiosity, acts in a confident way, shows a sense of humor, expresses hope and expresses sadness.
Regular conversation users are also considerably more likely than non-converses to say the main LLM they use responds in a brusque manner, embarrasses them, responds angrily and responds in a jealous way.
Policy issues
The Imagining the Digital Future Center and several other organizations have conducted surveys to gather public attitudes about some policy issues swirling around LLMs, such as their problems with generating wrong information and hallucinating; their problems with bias and discrimination; their environmental impact; and the opaque and mysterious ways of answering people’s prompts and queries. In this survey, we asked a question about which stakeholders should be mainly responsible for handling some of these issues. As a rule, these LLM users feel that the creators of LLMs themselves should be mainly responsible for addressing problems.
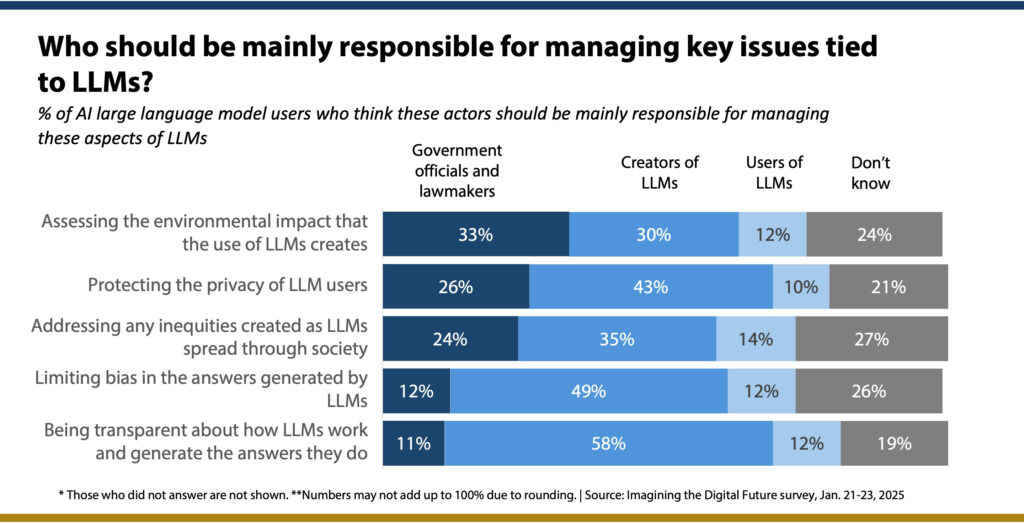
There are some partisan differences on these issues among LLM users. Those who are Democrats and the independents who lean Democratic are more than Republicans and leaners to say government officials should assess the environmental impacts that the use of LLM creates (42% vs. 25%). In addition, Democrats are more likely to say government officials should address any inequities created by LLMs (31% vs. 16%). While majorities of LLM users from both parties think the creators of LLMs should be responsible for being transparent about how LLMs work, Democrats are somewhat more likely than Republicans to pick government officials (15% vs. 8%). There are not partisan differences on the other issues listed here.
The longer-term impact of LLMs
These LLM users render mixed judgments about how these models will evolve and affect the quality of people’s daily lives in the future. More think the effect will be more positive than negative than think the opposite. Still, a third think there will be an equal mixture of positive and negative impacts, and 6% think there will not be much of an impact.
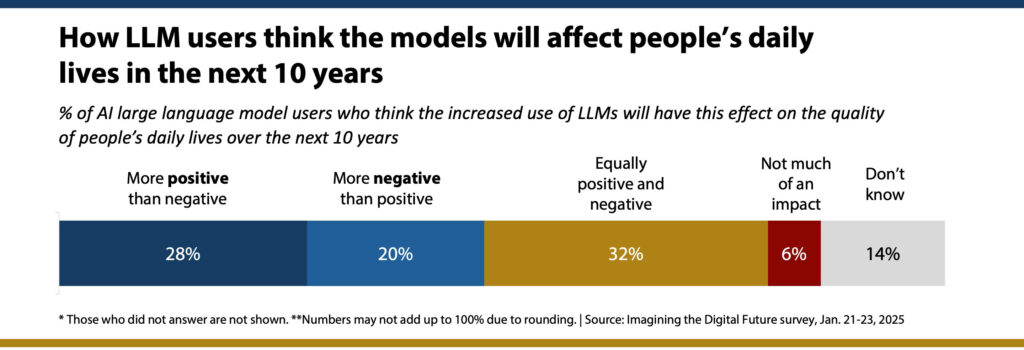
Asked about some more specific possible outcomes in the next decade, LLM users think these positive outcomes are very/somewhat likely to happen: major new medical and scientific breakthroughs and that LLMs will be controlled by human beings and responsive to human needs.
On the other hand, major segments of LLM users also think these negative outcomes are very/somewhat likely to occur as a result of the spread of LLMs in the next decade: social isolation, the loss of a significant number of jobs, LLMs will surpass human intelligence in most important ways, serious social upheaval will occur and they worry LLMs will develop their own identity and goals.
Notable shares of LLM users also predict some general impacts, including the fact that LLMs will considerably change the way they will spend their leisure time, will dramatically change the kind of work they do and will form deep relationships with people.
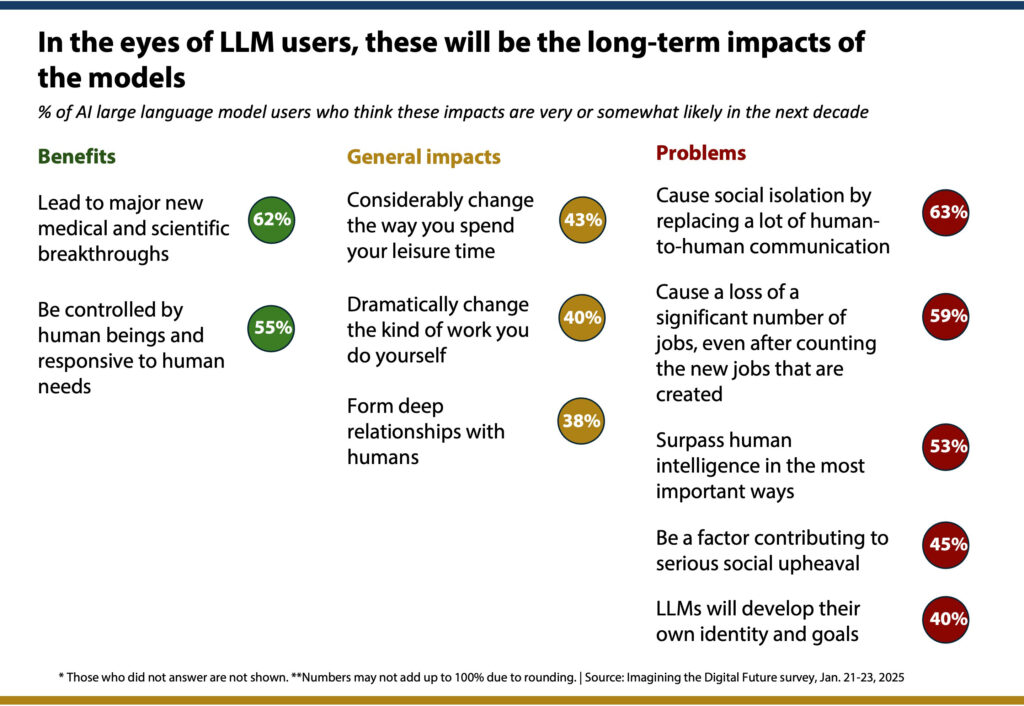